How to improve your data quality from online research
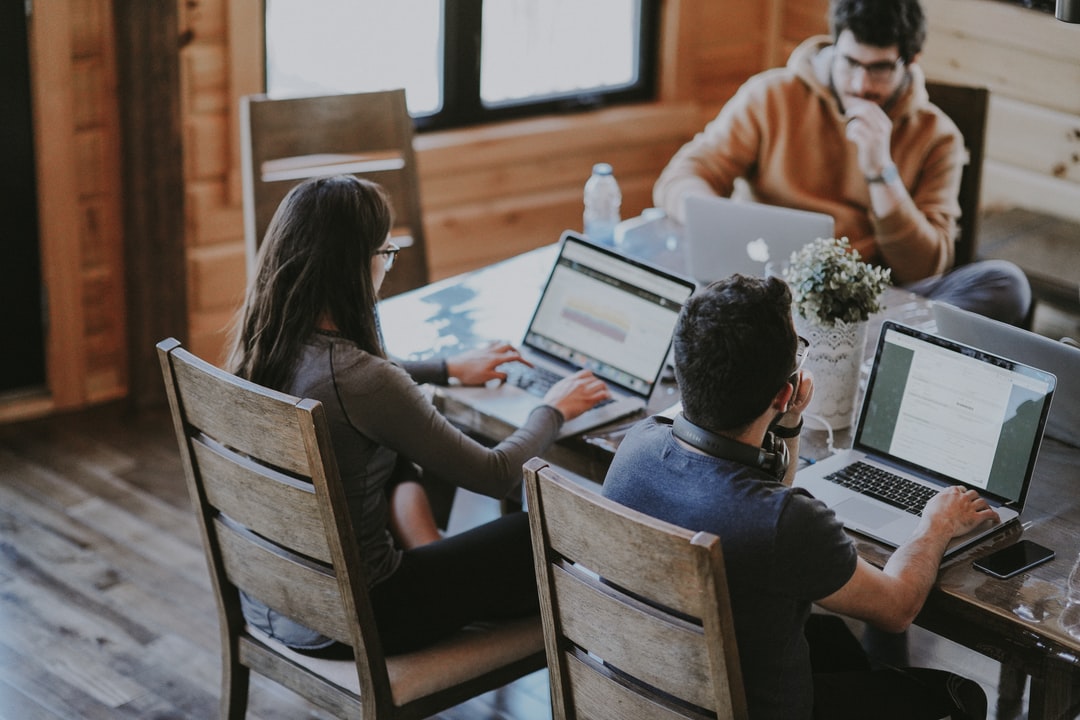
When it comes to ensuring data quality in online research, there are several factors you need to think about.
How do you know if your participants are paying attention? Are they doing the study properly? Are they who they say they are?
In this article, we’ll explore how to improve data quality in research online by busting bots, cracking down on cheaters, and stopping slackers from spoiling your data.
Bots, liars, cheats and slackers…
Malicious participants fall roughly into four groups: bots, liars, cheats, and slackers.
Bots
Bots are designed to complete online surveys with very little human intervention. They can often be spotted by their random, low-effort, or nonsensical responses.
However, bad-acting humans can prove trickier to detect...
Liars
Liars (or to use the technical term: malingerers) submit false pre-screening information. They do this to access as many studies as possible and maximise how much they can earn.
How badly can this mess up your data? That depends on two things:
- How important pre-screeners are to your study design: Let’s say you’re comparing male and female respondents. If some people in each group aren’t who they claim to be, this could invalidate the comparison and you can’t use the data. If sex isn’t a factor in your study design, then it doesn’t matter if a respondent lies about it or not.
- How niche your sample is: Impostors are more common when recruiting rare populations. This is because the competition for study places is quite fierce. Studies with broad eligibility fill up fast. So, if participants want to maximise earnings, they’ll need to access studies that fill more slowly by claiming to be a member of one (or many) niche demographics.
Cheats
These are participants who deliberately submit false information to your study.
Cheaters don’t always mean to be dishonest. ******Some may be confused about the data you’re trying to collect, or whether they’ll get paid even if they don’t do “well”. This can happen because they fear your study’s rewards are tied to their performance. They think they’ll only get paid if they achieve 100% on a test, so they Google the correct answers.
Or they might think you only want a certain kind of response (i.e., always giving very positive/enthusiastic responses). Or they might use aides (pen and paper) to artificially perform much better than reality.
The final kind of cheats are participants who don’t take your survey seriously. They might complete it with their friends, or while drunk.
To clarify: Liars provide false demographic information to gain access to your study. Cheats provide false information in the study itself. A participant can be both a liar and a cheat, but their effects on data quality are different.
Slackers
The fourth group are slackers. These people aren’t paying attention and aren’t interested in maximising their earnings. They don’t feel motivated to give you any genuine data for the price you’re paying.
Slackers encompass a broad group. They could be anyone from participants who don’t read instructions properly to people doing your study while watching TV. They may input random answers, gibberish, or low-effort free text.
Slackers aren’t always dishonest. Some may just consider the survey reward too low to be worth their full attention.
These groups do overlap. A liar can use bots, slackers can cheat, and so on. Most bad actors don’t care how they earn rewards, so long as they’re maximising their income!
So, what can you do about it?
Boosting your data quality
The tips below aren’t exhaustive. But they will give you some practical advice for designing your study and screening your data that will boost your confidence in the responses you collect.
Busting the bots
- Include a CAPTCHA at the start of your survey to stop bots from submitting answers. Equally, if your study involves an unusual interactive task (such as a cognitive task or a reaction time task), then bots should be unable to complete it convincingly.
- Include open-ended questions in your study (e.g., “What did you think of this study?”). Check your data for low-effort and nonsensical answers to these questions. Typical bot answers are incoherent and you may see the same words being used in several submissions.
- Check your data for random answering patterns. There are several techniques for this, such as response coherence indices or long-string analysis.
- Looking for a simpler solution? Include a few duplicate questions at different points in the study. A human will provide coherent answers. But a bot answering randomly probably won’t give the same answer twice.
Lassoing the liars
- Don’t reveal in your study title or description what participant demographics you’re looking for. It may give malingerers the information they need to lie their way into your study.
- Re-ask your pre-screening questions at the beginning of your study (and at the end too if it’s not burdensome). This allows you to confirm that your participants' prescreening answers are still current and valid. And it may reveal liars who have forgotten their original answers to pre-screening questions.
- Ask questions that relate to your pre-screeners that are hard to answer unless the participant is being truthful. So, if you need participants who take anti-depressants, ask them the name of their drug and the dosage. A liar might look up an answer to these questions, but most liars won’t be bothered to invest the extra effort!
Cracking down on cheats
- Use speeded tests or questionnaires, so participants don’t have time to Google for answers.
- Ask participants a few questions to clarify the instructions of the task at the end of the experiment. This is to check they understood the task and didn’t cheat inadvertently.
- Develop precise data-screening criteria to classify unusual behaviour. These will be specific to your experiment but may include:
- Variable cut-offs based on inter-quartile range.
- Fixed cut-offs based on ‘reasonable responses’ (consistent reaction times faster than 150ms, or test scores of 100%).
- Non-convergence of an underlying response model.
Simple as it seems, it’s been suggested you have a free-text question at the end of your study: “Did you cheat?”
No matter how you clean your data, we strongly recommend that you preregister your data-screening criteria. This will increase reviewer confidence that you haven’t p-hacked.
Sniffing out slackers
- Use speeded tasks and questionnaires so participants don’t have time to be distracted by the TV or social media.
- Ask participants a few questions to clarify the instructions of the task at the end of the experiment, to check they read them properly.
- Collect timing and page-view data by recording the time of page load and timestamp for every question answered.
- Record the number of times the page is hidden or minimised.
- Monitor the time spent reading instructions. Look for unusual patterns of timing behaviour: Who took 3 seconds to read your instructions? Who took 35 minutes to answer your questionnaire, with a 3-minute gap between each question?
- Implement attention checks (such as Instructional Manipulation Checks or IMCs). These are best kept super simple and fair. “Memory tests” are not a good attention check. Nor is hiding one errant attention check among a list of otherwise identical instructions!
- Include open-ended questions that require more than a single-word answer. Check these for low-effort responses.
- Check your data using careless responding measures. For example, consistency indices or response pattern analysis.
How Prolific keeps bots and bad actors out of your research
Does this all seem quite overwhelming? Don’t worry - when you use Prolific, our careful vetting measures and rigorous monitoring of our pool mean you won’t have to worry about banishing bots and bad actors.
We go to great lengths to ensure you connect with participants who are honest, attentive, reliable - and 100% human.
The initial checks
Firstly, we confirm that a participant is who – and where – they say they are. For every person who enters our pool, we verify their:
- Email address
- Phone number
- Photo ID
- PayPal address
A participant doesn’t start getting studies until we’ve verified the first three things on this list.
The ISP and VPN checks
We also check for some more technical things, like:
- IP addresses
- The Internet Service Provider (ISP)
- Device and browser information
- People using a VPN or proxy
We have a strict list of trusted ISPs. Some ISPs have a high risk of VPN and/or proxy usage. People can use VPNs and proxies to browse the internet anonymously. So, we don’t allow it on Prolific.
It’s critical that our participants are who they say they are.
The first test
Once we verify a participant, we invite them to complete a test study. Here, we check them for attention, comprehension, and honesty. The study requires them to write a short story about superheroes. If the story is meaningful and makes sense, they can do more studies.
The results of this approach speak for themselves. In an independent study, Prolific participants showed low levels of disengagement and were found to be more attentive compared to participants on MTurk.
The ongoing checks
When you approve a study, then the participant gets paid and you get the quality data you need.
If you reject a study, however, then that gets recorded on the participant’s account. Too many rejections, and they can’t do any more studies.
We also use an algorithm called Protocol to perform ongoing checks on the platform. This helps identify bots and bad actors that abuse the system – and take studies away from our thousands of real and committed participants.
Feedback
If you’re finding the data from some participants to be unusable or suspect something fishy going on with duplicate accounts, you can report them to us. You can do this with our in-app reporting function, or via our support request form.
We then review the account and decide if a ban is suitable. Of course, feedback is a two-way street. Participants can also report researchers if they feel they’re not being treated fairly.
These measures ensure you get high-quality data from a panel of the most honest, attentive, and reliable participants.
Getting the best out of Prolific participants
A critical factor in determining data quality is the study’s reward. A recent study of Mechanical Turk participants concluded that fair pay and realistic completion times had a large impact on the quality of data they provided.
On Prolific, that trust goes both ways. Properly rewarding participants for their time is a large part of that. We enforce a minimum hourly reward of £6/$8. But depending on the effort required by your study, this might not be enough to foster high levels of engagement and provide good data quality.
Consider:
- The guidelines of your institution. Some universities have set a minimum and maximum hourly rate (to avoid undue coercion). You might also consider the national minimum wage as a guideline.
- How much effort your study requires. Is it a simple online study, or do participants need to make a video recording or complete a particularly arduous task? If your study requires more effort, consider paying more.
- How niche your population is. If you’re searching for particularly unusual participants (or participants in well-paid jobs), then you will find it easier to recruit these participants if you are paying well for their time.
Error-free, clear, and engaged
Ultimately, participants are responsible for the quality of the data they provide. But you as the researcher need to set them up to do their best.
- Pilot your study's technology. Run test studies, double and triple-check your study URL, and ensure your study isn’t password-protected or inaccessible. If participants find errors, they’ll plough on and try to do their best regardless. This may result in unusable or missing data. Don’t expect your participants to debug your study for you!
- Keep your instructions as clear and simple as possible. If you have a lot to say, split it across multiple pages. Use bullet points and diagrams to aid understanding. Explicitly state what a participant needs to do to be paid. This will increase the number of participants that do what you want them to!
- Think about participants who will be seeing your research for the first time, or speak English as a second language. It might take them longer to digest the content of your survey. Factor this in when you estimate how long your study will take to complete. For instance, you could try working through the survey yourself at a slower pace.
- If participants message you with questions, try to respond quickly and concisely. Be polite and professional (it’s easy to forget when 500 participants are messaging you at once that each one is an individual). Ultimately participants will respond much better when treated as valuable scientific co-creators.
- If you can, make your study interesting and approachable. Keep it easy on the eye and break long questionnaires down into smaller chunks.
- If you can, explain the rationale of your study to your participants. There is evidence that participants will put more effort into a task when its purpose is clear, and that participants with higher intrinsic motivation towards a task provide higher-quality data.
What to do if you think your data quality is compromised
Firstly, talk to us. Reject submissions where you think this has happened and send us any evidence you’ve gathered. Data quality is our top priority, so please reach out to us if you have any concerns, queries, or suggestions.
In cases of cheating or slacking, we ask that you give participants some initial leeway. If they’ve clearly made some effort or attempted to engage with the task for a significant period but their data isn’t good enough, then consider approving them, but excluding them from your analysis. If the participant has clearly made little effort, failed multiple attention checks, or has lied their way into your study, then rejection is appropriate. Please read our article on valid and invalid rejection reasons for more guidance.
You can also learn more about how to improve data quality in research in The Complete Guide to Improving Data Quality in Online Surveys. You’ll discover:
- Common data quality issues.
- Tried and tested ways to improve the data quality from your online survey responses.
- How Prolific can help you achieve high data quality.