The complete guide to selection bias
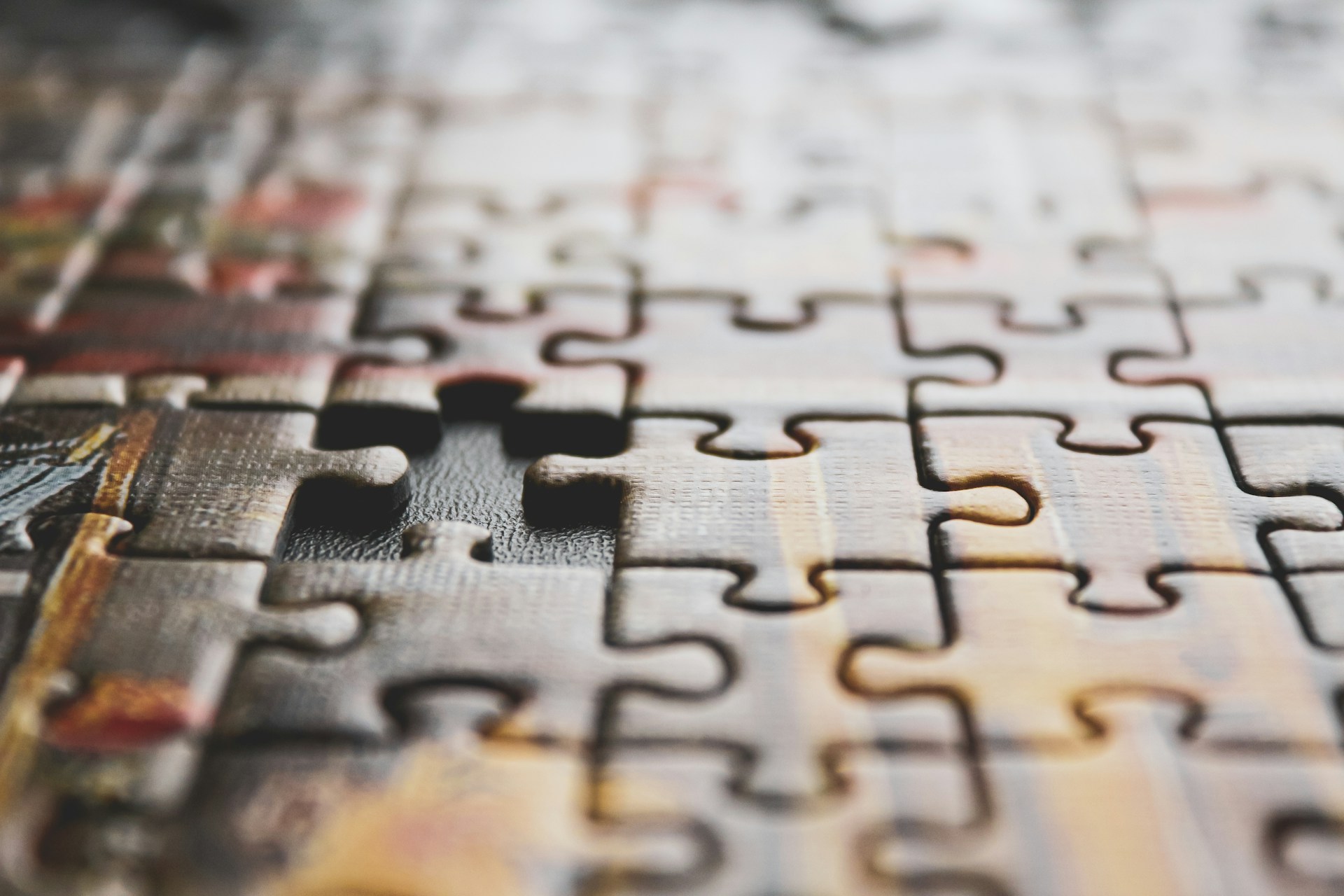
As the old saying goes, knowledge is power. But the quest for knowledge isn’t always as easy as we’d like it to be.
Researchers often find themselves delving into datasets, dissecting information, and uncovering insights that can shape entire fields of study. However, amongst the excitement of new discovery lies a subtle yet complicated obstacle: selection bias.
This phenomenon has the potential to warp conclusions, skew perceptions, and cast doubt on the integrity of your findings. But what is it, and how can you avoid it in your own research? Let’s take a look.
What is selection bias?
Also known as the selection effect, selection bias occurs when a sample used in a study isn’t completely representative of the population of interest or is sub-optimal for answering the specific research question. This could be introduced through different sampling methods or the way the participants were selected. Or it could just come down to the particular area of interest being researched.
This bias then distorts the results of the study, undermining its value and rendering it untrustworthy.
There are a variety of different types of bias, each bringing their own implications. These are:
1. Sampling bias
Sampling bias occurs when certain members of the population of interest have a higher or lower chance of being selected than others. When this happens, the research won’t give a very representative point of view.
2. Survivorship bias
Survivorship bias is when only successful subjects are included in the final analysis, leading to a skewed outcome. This is often seen in studies of successful people or companies, where failures are taken out of the equation.
3. Self-selection bias
Self-selection bias is where people nominate themselves to be part of a study, leading to a non-random sample of participants. This is often prevalent in surveys or online polls, where the people who take part may not represent the population as a whole.
4. Information bias
Information bias happens when there are systematic errors in the measurement or collection of data. This makes the outcomes just as unreliable.
5. Non-response bias
There will also be people who refuse to take part in or drop out of a study. It’s likely that there will be some kind of underlying commonality in these participants. For example, they might be male, or under the age of 20.
Examples of selection bias
To understand the more practical implications that selection bias can have on a study, let’s take a look at some real-life scenarios.
1. Clinical trials
Clinical trials can see a significant impact from selection bias, largely due to self-selection bias. This can impact the effectiveness of the drug being trialed. For example, where younger people are more likely to take part in clinical trials, the results may only show the impact in that age group. So, older people aren’t represented.
2. Job recruitment
A common selection bias example is recruitment. Hiring processes can also fall prey to selection bias. If your company relies on employee referrals as one of your main recruitment methods, this can mean that individuals from different backgrounds or networks are excluded from the process entirely. This may result in a workforce that lacks diversity and unique thinking.
3. Economic studies
These can be vulnerable to survivorship bias. Especially in studies of successful businesses or investment strategies. For example, if a study only examines companies that have achieved significant growth, it may overlook the failures and challenges faced by less successful enterprises.
Survivorship bias distorts perceptions of risk and reward. This can lead to flawed investment decisions.
4. Educational research
In educational studies, selection bias can distort assessments of teaching interventions or educational programs. For example, you might want to look at the effectiveness of a tutoring program. But if you only include students from affluent areas, the findings will likely be irrelevant to students from disadvantaged backgrounds.
The impact of selection bias
Why is selection bias so concerning? Because it can result in misleading conclusions and send researchers down the wrong path. And when this happens, outcomes don’t align with reality.
The ramifications of selection bias extend beyond just the statistics. They can also result in wasted resources. Valuable time, money, and manpower are used up on research that doesn’t reflect the population of interest. This can stop progress and have negative or unfair impacts on certain groups of people.
It can also lead people to lose trust in science. When research comes across as biased or unfair, it makes people doubt if science can really help us. This can have hugely negative impacts on society as a whole.
How to avoid selection bias in your own research
To get accurate results and draw meaningful conclusions, you need to conduct research that's fair and minimizes bias. But how?
Here are some simple yet effective strategies to ensure you conduct research with integrity and impartiality:
1. Define your population
Clearly define the population you want to study and make sure you understand who should be included and excluded from your research. Let's say you want to gauge people's understanding of a new financial services product. You would define your population of interest (people who would use that product), then ensure your sample reflects that population.
2. Random sampling
Use random sampling to select participants from your population. Imagine you're conducting a survey on public opinion about a controversial social issue. Instead of selecting participants based on convenience or availability, use random sampling to ensure that every member of the population of interest has an equal chance of being included in your study. This helps reduce the risk of bias and ensures that your findings are representative of the population as a whole.
3. Stratified sampling
If your population has different subgroups, you can use stratified sampling to ensure representation from each group. By sampling randomly within the strata, you can capture the diversity of your population more accurately. This avoids biases introduced by over or underrepresentation of certain groups.
4. Minimize exclusions
Try to reduce as many exclusions as possible from your study unless absolutely necessary. Excluding certain groups or individuals can introduce bias and limit the effectiveness of your findings.
5. Transparent reporting
Be transparent about your selection process in your research reports. Clearly document how participants were selected, as well as any criteria used for exclusion. This information gives people a clear insight into your methodology. And it helps readers to build trust with your findings.
6. Consider alternatives
Explore alternative methods of data collection or sampling if traditional methods introduce bias. For example, if you're carrying out a study on consumer preferences for a new product, consider using a combination of online surveys and focus groups to reach a diverse range of participants. This approach helps reduce bias by relying solely on one method of data collection and ensures that your findings are robust and reliable.
7. Consult experts
Seek input from colleagues or experts in your field to review your research design and selection process. Fresh perspectives can help identify potential sources of bias that may have been overlooked, enhancing the credibility of your research and ensuring you offer diverse viewpoints and methodologies.
How Prolific can help
At Prolific, flexibility and control are right at the heart of everything we do. With our pool of 120,000+ active participants, all fully vetted and verified, you can rely on us to deliver definitive and varied data sets, no matter what your research topic is.
Sign up to Prolific today to gather balanced, representative samples for your research.