How to avoid bias in research: 15 things marketers need to know
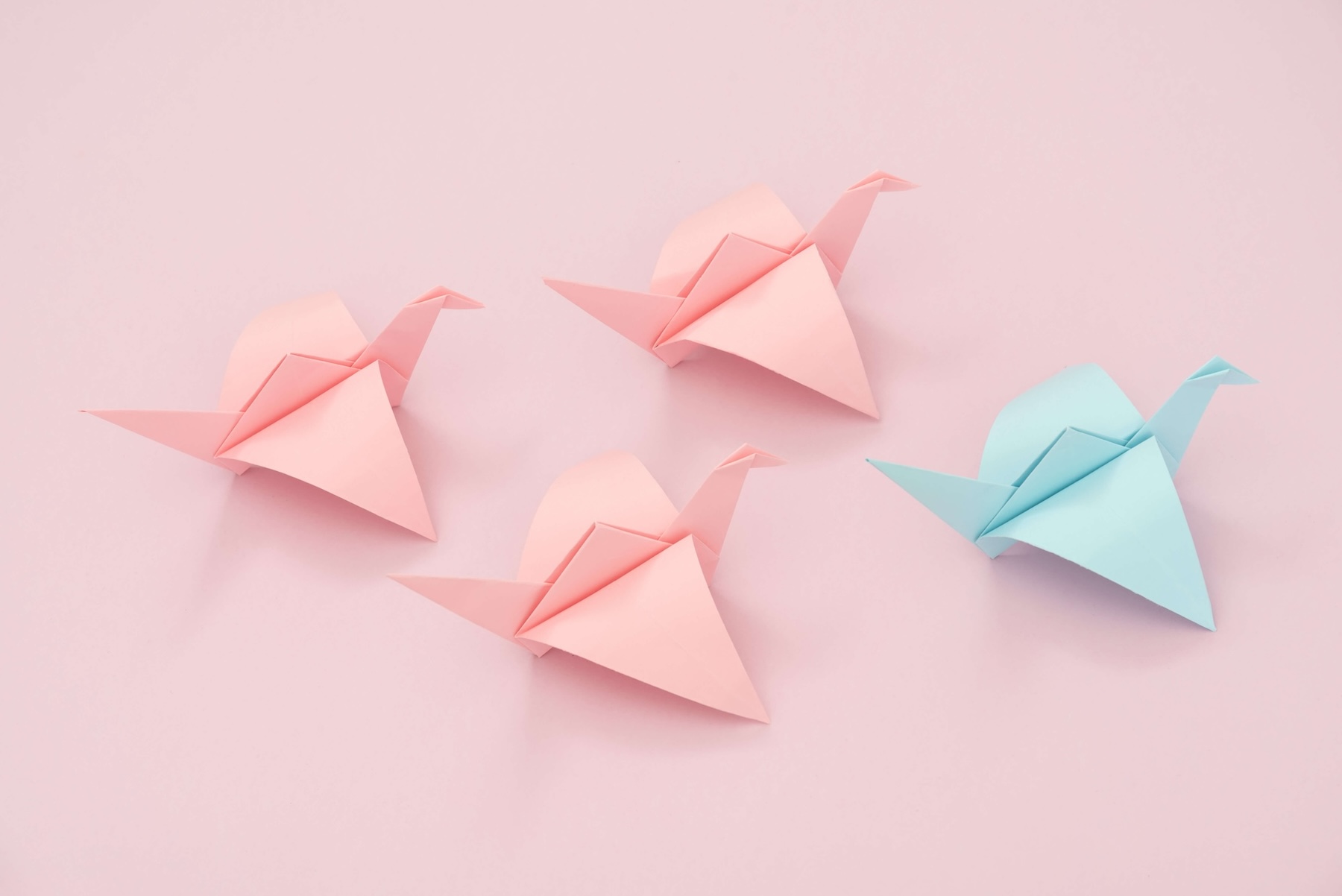
1. Beat selection bias by gathering diverse participants
Selection bias happens when your survey sample is skewed, which will inevitably result in data that doesn’t give you the full picture. This bias takes multiple forms, each of which can result in your team overemphasizing certain viewpoints at the expense of others.
Sample size bias is caused by surveying too small an audience. This error returns results that don’t generalize to the wider population. Inclusion criteria bias happens when the survey audience doesn’t represent the population as a whole. Researchers who only talk to those who are easy to contact, or ignore part of their audience, will get results that over- or under-represent certain personality types.
Avoid this bias by determining the minimum viable sample size for your survey. Then, gather a representative sample of your target audience. Your ideal audience will depend on the goals of your study. If you want to speak to “purchasers of HR tools at small and medium businesses,” it’s not problematic to leave out professionals at enterprise companies. Just be aware that the data you gather from your SMB respondents can’t be extrapolated to enterprise workers later.
Prolific makes it easy to find an audience that matches your criteria without over- or under-sampling subpopulations. See how it works with a free account.
2. Do a practice run to spot hints of procedural bias
Procedural bias refers to the ways responses might be influenced by the circumstances and administration of your study. One of the most common causes of procedural bias is setting a time limit for your study. Participants may rush their answers if they know their time is limited or, worse, can see the time left counting down as they go.
Participants’ answers may be influenced by their setting - for example, if they’re in a loud room where they cannot properly hear a video, or if their internet is too slow to download images. The platform you use also matters. A tool that doesn’t work well on phones will likely give you fewer answers as a whole and may exclude younger or lower-income participants.
Online surveys put the participants in control of their setting, but you can minimize the effects of this bias by suggesting a certain environment (e.g., “We recommend you take this survey on a laptop in a quiet room”). Make sure your questionnaire follows accessibility best practices and is optimized for mobile use to remove platform bias. Finally, it’s a good idea to test your survey with people who aren’t involved in the design process and won’t be final respondents to see if there are any outstanding procedural barriers.
3. Avoid leading questions and other types of wording bias
Wording bias can subtly influence respondents toward giving answers that affirm your expectations. Improperly worded questions tend to contain assumptions.
For example, asking, “What kinds of foods do you eat for breakfast each morning?” presupposes a respondent has breakfast every day and may obscure the fact that many Americans don’t. Leading questions presume they already know a participant’s answer. Consider the difference between “Do you wish you ate breakfast more?” and “How satisfied are you with your breakfast schedule?” The first is clearly looking for a “yes,” whereas the second doesn’t suggest how a respondent should feel.
Avoid wording bias by asking yourself what answers you’re hoping for and then looking for subtle cues or nudges in your questions. Research bias isn’t the reason moviegoers have been unimpressed with Sony’s attempt at its own Marvel franchise. But it was the reason for a weekend during which its 2022 title Morbius earned an embarrassing $280 per theater.
Most studios would see a film’s poor critical reception and failure to recoup its budget as signs that a movie didn’t meet viewers’ expectations. Not Sony. The studio’s attention was stuck on Twitter, where Morbius trended for weeks after its release thanks to the impressive traction of Morbius memes.
Execs, excited by the attention, planned a special weekend re-release of the film. It returned to 1,000 theaters across the U.S…and made just $280,000.
Multiple types of research bias contributed to this embarrassing mistake. First: selection bias. They treated Twitter’s trending topics as representative of American consumers’ interests rather than representative of a relatively small group of individuals. Second: confirmation bias. When Morbius trended, they assumed the attention meant people would want to see it in theaters. Third: misclassification. Somehow, no one determined that the memes poking fun at the movie were, in fact, bad press.
Marketers should learn from Sony’s mistakes so they don’t follow suit. Many types of bias can affect market research; all lead to the same thing: bad data, and therefore, bad business decisions.
Here are some common types of research bias — and how to prevent them. Learn them before your next survey, so you can trust your data and make decisions with confidence.
Design bias
Your methodology can affect the outcome of your study. These biases get more difficult to spot and remove the further you get into your study because they become part of the way things work. Be aware of study design decisions that can influence participant answers and make your data less accurate.
Interviewers should never state implied feelings and should use a participant’s own language (“You said you sometimes eat breakfast. Can you define that?”) rather than pre-written text (“If you don’t eat breakfast every day, do you eat it often?”)
4. Know how question order effects can change participant answers
Order effect bias shows up when participants use your questions to build context for each other and adjust their answers accordingly. Participants are likely to fall into the groove of behavioral consistency. They’ll want to give answers that uphold their previous opinions.
Imagine one of your first questions solicits opinions on the RiteAway Smooth Gel Ink Pens. If a few questions later, you ask about the RiteAway brand, people are likely to answer the second question in a similar way to the first because they want to seem consistent.
Randomizing question order is the best way to avoid this bias. While individual participants may draw connections between questions, they’ll all do so in different ways, so your data as a whole will remain viable. Surveys that won’t work with randomized data should ask:
- General questions (“How often do you purchase office supplies for your company?”) before specific questions (“How often do you purchase ink pens for your company?”)
- Unaided questions (“Please name three brands of ink pens”) before aided questions (“Have you heard of RiteAway Ink Pens?”)
- Positive questions (“What do you like best about the ink pens your company purchases?”) before negative questions (“What is one thing the ink pens your company purchases could do better?”)
This is another type of bias that may be easiest to see by testing your questions on individuals who won’t be participating in the study. Ask to hear the thought process behind each answer — your testers may even tell you when a previous question has influenced their response.
Researcher bias
Researcher bias colors data when the individuals administering your study let their own opinions, worldview, and behaviors affect the data they gather. Any study in which researchers interact directly with participants must be preceded by training. Respondent-facing individuals should be aware of their body language and tone of voice at all times and have both under control. You may also want to set up cameras or audio recorders so you can review sessions for actions that may have affected participants’ responses.
Online surveys are much less likely to contain researcher bias because they remove interpersonal factors. However, you must still beware of how individual biases can sneak in during the setup and analysis phases.
5. Educate interviewers to reduce the risk of cultural bias
Cultural misunderstandings between interviewers and their subjects can impede accurate data collection. Everyone’s understanding of the world (and others’ behaviors) is influenced by the values and standards of their culture. One place this shows up is our language: “Looks Gucci” and “that’s sick” are two very different ways of expressing a similar sentiment.
Your researchers should understand cultural differences they may encounter before administering any surveys. That means learning about community practices and norms that apply to your subjects. They must then do their best to understand a respondent’s answers in the context of those practices and norms rather than their own. Even when they don’t understand, they should meet each response with positivity and a lack of judgment.
6. Separate questions by topic to suppress the halo effect
The halo effect (and its counterpart, the horn effect) can lead researchers to extrapolate subjects’ feelings and miss nuances. For example, if an individual is well-groomed, prompt, and professional, the researcher may assume they’re engaged and answering honestly. They may be less likely to ask follow-ups or note inconsistencies — after all, a trustworthy individual wouldn’t say anything contradictory.
This form of bias can also affect how a researcher understands answers to related questions. If a respondent expresses love for the carmaker Vitesse, the researcher may assume that enthusiasm also applies to later questions about individual Vitesse models. They might then downplay the participant’s frustration at their Vitesse Frontière’s low gas mileage.
Mitigate the halo effect by grouping questions by brand or subject and finishing each topic before moving on to the next. Clearly demarcate the change to help curb a researcher’s impulse to carry respondent sentiments forward.
7. Record all answers in the same manner to avoid confirmation bias
Confirmation bias makes researchers more likely to remember and record data that confirms their worldview. Imagine a product comparison wherein your interviewee says your brand — Hikrr — seems fun and trustworthy, but they also express loyalty to REI. An in-house researcher who didn’t fight this bias would downplay or even forget the positivity around REI because they personally believe you have superior products.
Fight this type of bias by using unaffiliated researchers who are naïve to your field or study — meaning they have no previous experience with it and, therefore, fewer preconceptions. If you cannot do so, instruct your researchers to record all data rather than just the things that “seem important.” Keep in mind that any researcher may still bring biasing worldviews to their work. They should follow the same strict reporting rules as affiliated researchers would.
Respondent bias
People aren’t always honest, especially during conversations with someone they don’t know. A big part of getting useful data is making it clear you value honesty, respect participants, and are there to learn from them without judgment.
It’s impossible to avoid all types of participant bias because you can’t control your subjects. You can minimize it by crafting a process and questions that make forthrightness easier.
8. Balance your questions to overcome acquiescence bias
The urge to agree with statements causes acquiescence (or friendliness) bias and can distort your data with overwhelmingly positive responses. Acquiescence bias can also be a sign of a too-long survey; respondents often just say “yes” to everything to speed up the process. No matter the cause, you’ll end up overestimating consumer sentiment.
Since people are more likely to say yes to any question, you need to make sure your survey is neutral or balanced. Neutrality means asking questions that don’t have a yes/no answer, such as “Using the scale below, how do you feel about Lauren’s Breads?” Include reverse-coded items such as: “Agree or disagree: I am likely to visit Lauren’s Breads in the next month.” and “Agree or disagree: I am unlikely to visit Lauren’s Breads in the next month.”
9. Vary your question structure to avoid habituation bias
Subjects may also “yes” their way through a survey if they’ve disengaged because every question is worded similarly. That behavior is called habituation bias. Users may respond “yes” to 10 questions in a row if, for example, they each start with “Do you like…?”
Exchanges that feel conversational are the most effective hedge against habituation bias. Do your best to vary the question format and structure to prevent respondents from answering reflexively. Prolific also allows surveys that include attention checks to identify anyone answering on autopilot. These questions make it easier to weed out bad data.
10. Avoid brand or company affiliations, or you'll see sponsorship bias
Interviewees want your research to succeed, and sponsorship bias shows up when they figure out your goals and try to answer in the “right” way. Respondents who identify the company behind the survey may rate that brand or its products more favorably. Those who know your brand’s mission or values may try to show how much they align with them.
Combat this bias by keeping information about your study confidential. Your recruitment process, informational materials, and researchers shouldn’t have any clear affiliation with your brand. Each survey should start with a statement of independence and neutrality, and you should never share the goal of your study or its sponsors, if possible.
11. Combat social desirability bias by making it okay to go against the flow
Our desire to fit in leads to social desirability bias, which causes respondents to give answers they think will make their interviewer like and accept them. Social desirability bias is an especially strong force when you’re asking about sensitive topics. Questions about alcohol use, for example, are likely to receive answers that underestimate the amount people drink and the frequency of binge drinking.
Preface such questions with a statement that protects the subject’s privacy (“all answers will remain anonymous”), or use a tool like Prolific that keeps them anonymous to encourage honesty. You can also ask indirect questions (“Do you think a typical employee has lied at work because they didn’t want to look bad in front of their boss?”) instead of direct ones (“Have you ever lied at work because you didn’t want to look bad in front of your boss?”). This practice allows respondents to protect their reputations by ascribing the behavior or opinion to a hypothetical third party.
Bias during analysis and reporting
Even the best data can lead to wrong conclusions if a researcher’s assumptions are coded into the results. Reducing bias during analysis requires your team to be aware of any assumptions they hold. You should have strict guidelines in place to prevent these biases from coloring data interpretation.
12. Include all data in your first analysis to prevent p-hacking
Don’t filter data before you begin your analysis, lest you accidentally engage in p-hacking. Excluding responses based on an in-the-moment rationale may leave you with cherry-picked results that over- or under- emphasize certain viewpoints. You’re likely to end up missing context or nuance from your final report.
Keep your results accurate by creating exclusion criteria before you even start your study and holding your team accountable to them. Your team should code and analyze all the information that doesn’t fall afoul of those pre-set standards.
13. Gather multiple interpretations to avoid misclassification
Have at least two people code the same set of data (in isolation from one another) to see if they interpret responses the same way. Researchers who are tasked with interpreting survey results might misclassify data based on individual assumptions or cultural differences. Say a respondent answered, “Yeah, no,” when asked if they planned to return to their pre-pandemic frequency of dining in restaurants. A researcher might interpret that as a yes, a no, or indecisiveness depending on their reading and familiarity with that phrase.
Measure inter-rater reliability to determine the reliability of your researchers’ assessments. If their interpretations don’t match at least 75% of the time, you’ll need to determine what caused the differences and re-code your data.
Divergent answers may be caused by cultural differences or underlying assumptions. You may be able to tell what caused the disconnect and which interpretation is correct just by looking at the data and both results after. If you can’t, ask both individuals to explain their reasoning.
Situations where the data itself is unclear are best fixed by asking the subject. Prolific has a built-in messaging system to make this type of communication easy. If you’re using another tool, make sure you ask for permission to follow up with participants and gather their contact info for this purpose.
14. Compare your results to others, but beware of publication bias
It’s wise to verify your results by comparing them to similar studies, though publication bias may lead to a skewed public viewpoint. Outcomes that don’t meet a sponsor’s goals, which disprove a hypothesis, or that show a null effect are all less likely to make it to publication.
There’s no way to “fix” this bias since it’s caused by others’ actions, but you can work around it. First, make sure you search for a variety of viewpoints on your topic. Then, take note of each study’s sponsors. Do studies set up by industry groups show one result while academic studies come to a different conclusion?
You should also look at a study’s methodology to see if you can find any signs of bias. Many things that could affect results won’t be recorded, but if you see a problematic element, you’ll know the study might not be reliable. It may be worth revisiting your own process before publishing if you find yourself in agreement with a clearly biased study.
15. Get an outside opinion to catch bias you missed
Ask someone from a different industry or background to double-check your work for hidden assumptions or flawed logic. Try to keep the individual naïve to the goals of your study if possible. If they don’t share your expectations, they’ll have an easier time spotting data or interpretations that seem forced. They may also be able to spot gaps in your reasoning.
If your reviewer catches bias that stemmed from the design, researchers, or respondents, you won’t be able to do anything to fix it at this point. The only solution is a new study that follows better practices. If bias slipped in during the analysis and reporting phase, you could simply repeat that phase after fixing your practices.
Give everyone the tools to assess your study fairly
Let your readers know how you conducted your research study so they can determine for themselves whether to trust the results. If your data isn’t proprietary, consider publishing it on OSF so others can do their own analyses. You may also want to point out questions you didn’t get a satisfactory answer to and want to research further. This won’t undermine your findings. Your self-awareness will increase credibility by showing readers that you put thought into your work. People who claim to have all the answers are usually lying to you; the same goes for studies.
Just as research is about the pursuit of knowledge, a researcher’s job is not to know things but to learn things. Your understanding of how bias can find its way into research will help you better evaluate studies — your own and others’ — and improve the quality of information available to all.
From robust demographic screeners to anonymity protection to quality control features, Prolific has the tools you need to administer an unbiased survey. Sign up for free today!