Data quality at Prolific - Part 1: What is a "good participant"?
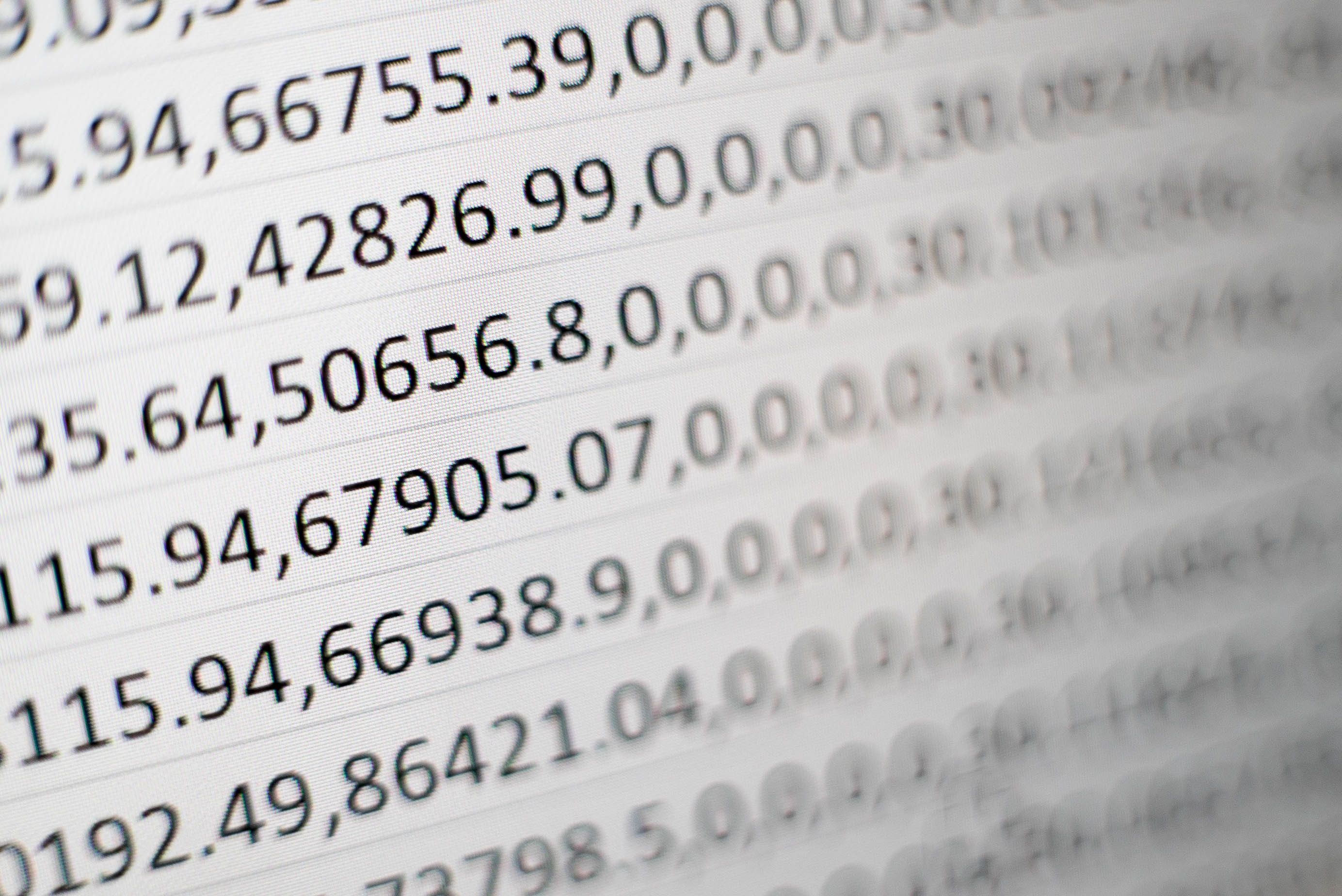
Data quality has been, and continues to be, a top priority at Prolific. We understand that without good quality data you can't conduct good research. Imagine having spent thousands from your research budget only to collect a heap of mediocre data at best and unusable data at worst! π¬
We've talked about data quality in the past, but we still have a lot more to say on the topic! This autumn, we're launching a miniseries about data quality at Prolific in three parts:
- Part 1: What is a "good quality participant?"
- Part 2: How do we regulate naivety and maintain engagement?
- Part 3: Using machine learning to assess attentiveness and monitor trustworthiness
Our goal with this miniseries is not simply to tell you about our approach to measuring and maintaining data quality on Prolific. As scientists, we know our system is not foolproof. We want to kick-start a broader discussion about what good data quality is, to hear your thoughts on how to measure it, and to gather ideas to help us ensure that Prolific is the most trustworthy place to conduct research online!
We raise some questions in this post: Please tweet us (@Prolific) your thoughts or comment below!
The NEAT framework
Because data quality is a top priority for us, we've spent a lot of time thinking about what it means for a participant to be "good quality". One of the tools we've created is something called the NEAT Framework (yes, it's a cheesy backronym, we know, we know π).
NEAT stands for Naive, Engaged, Attentive and Trustworthy: four attributes we think represent the ideal research participant. The NEAT framework has helped guide the development of all aspects of our data quality systems: from the initial automated reviews of a participant's trustworthiness, all the way through to the way we distribute studies.
Though all aspects of the NEAT framework are important, the concepts are organised from least fundamental to most fundamental. Let's get into the weeds of it:
Naive
Certain types of study depend strongly on participant naivety in order to obtain truthful results. If participants are familiar with the experimental manipulations used, are experts in the topic at hand, can guess the research question or have taken part in enough research that they can spot deception a mile off, then their responses may be biased as a result. As such, conclusions based on non-naive samples might not generalise well to the wider population.
Currently, participants on Prolific are less naive than your average person on the street (they have sought Prolific out after all π€·ββοΈ). But as we'll discuss in part 2 of this series, we have a number of mechanisms at our disposal for measuring and controlling naivety on our platform. Plus, rest assured that in 2020, we will tackle the question of participant naivety head on! Itβs on our roadmap to diversify our pool, which includes recruiting more βnaiveβ participants who take part in research sporadically rather than regularly.π
The concept of Naivety reflects a tension at the heart of the NEAT framework. Perfectly naive participants have never taken part in research before, so we know very little about how trustworthy they are. Plus, perfectly naive participants may be confused by some of the standard (and not so standard) elements of online research, such as consent forms, longitudinal studies, downloaded software, web-cam recordings, etc. As such, they are less likely to properly engage with the study process.
This means that it isn't possible for you to be confident that a participant is engaged, attentive and trustworthy and to say they are fully naive. Instead, we aim to strike a balance between all aspects of the NEAT framework.
What do you think is more important? Naive participants or trustworthy, engaged participants? When is one more important than the other?
Engaged
An engaged participant is sincere, motivated, and diligent. In other words, participants should complete each task/answer each question to the best of their ability. In addition, engaged participants are more likely to stick around for a longitudinal study, wrestle through technical difficulties, and to message researchers when they encounter an issue. These are people who care about the quality of the answers they give, and are willing to put in the effort to see it done well.
In contrast to naivety, participant engagement is something researchers have a relative control over. So in part 2, we'll talk in greater detail about engagement's relationship with reward per hour, good study design, and the ways in which Prolific seeks to both measure and improve it.
Attentive
Attentive participants pay attention to instructions so that they can provide meaningful responses. In many ways, attentiveness is similar to engagement. But where engagement is a spectrum and researchers might want to trade it off for increased naivety, attentiveness is a mandatory barrier which we believe all good participants must cross.
In part 3, we'll talk more about the ways we catch inattentive participants (and their effectiveness). But we also encourage all researchers to include attention checks in their studies (and have detailed guidelines on how best to do this). However, the goal of these checks must be to catch maliciously negligent participants, and not to trip up the unwary (or naive!).
Recently we've noticed some debate about our definition of fair attention checks, so we'd like to hear your perspective. π
What is the best way to implement attention checks, and what other methods do you like for ensuring that participants have read your questions properly?
Trustworthy
If you've used Prolific before, you'll know that we care a lot about participant anonymity. While we appreciate this might seem at odds with our goal of giving researchers transparency, we believe that anonymity is a big reason why participants trust us. Besides, recent years have shown us the risks of facilitating un-anonymised survey data collection...
Our job as custodian of participant anonymity, is to make sure that participants are who they say they are, so that you don't have to.
This means detecting and suspending people who try to operate multiple participant accounts, who misrepresent themselves in their prescreening information, and "people" who are bots. After all, if you don't trust the people you're surveying, you can hardly draw meaningful research conclusions.
This is why trustworthiness is the cornerstone of data quality work at Prolific, and this is why we're currently testing a new, more automated, system for monitoring our participant pool and finding fraudulent participants. We're using machine learning for this and we can't wait to tell you more about how this system works in part 3!
And that's a wrap for Part 1 of the miniseries.
What do you think about the NEAT framework β does our conceptual framework for data quality make sense to you?
Stay tuned to Parts 2 & 3, and don't forget to answer our questions above! π
Cheers,
Prolific Team
Come discuss this blog post with our community!