What is multi-modal AI?
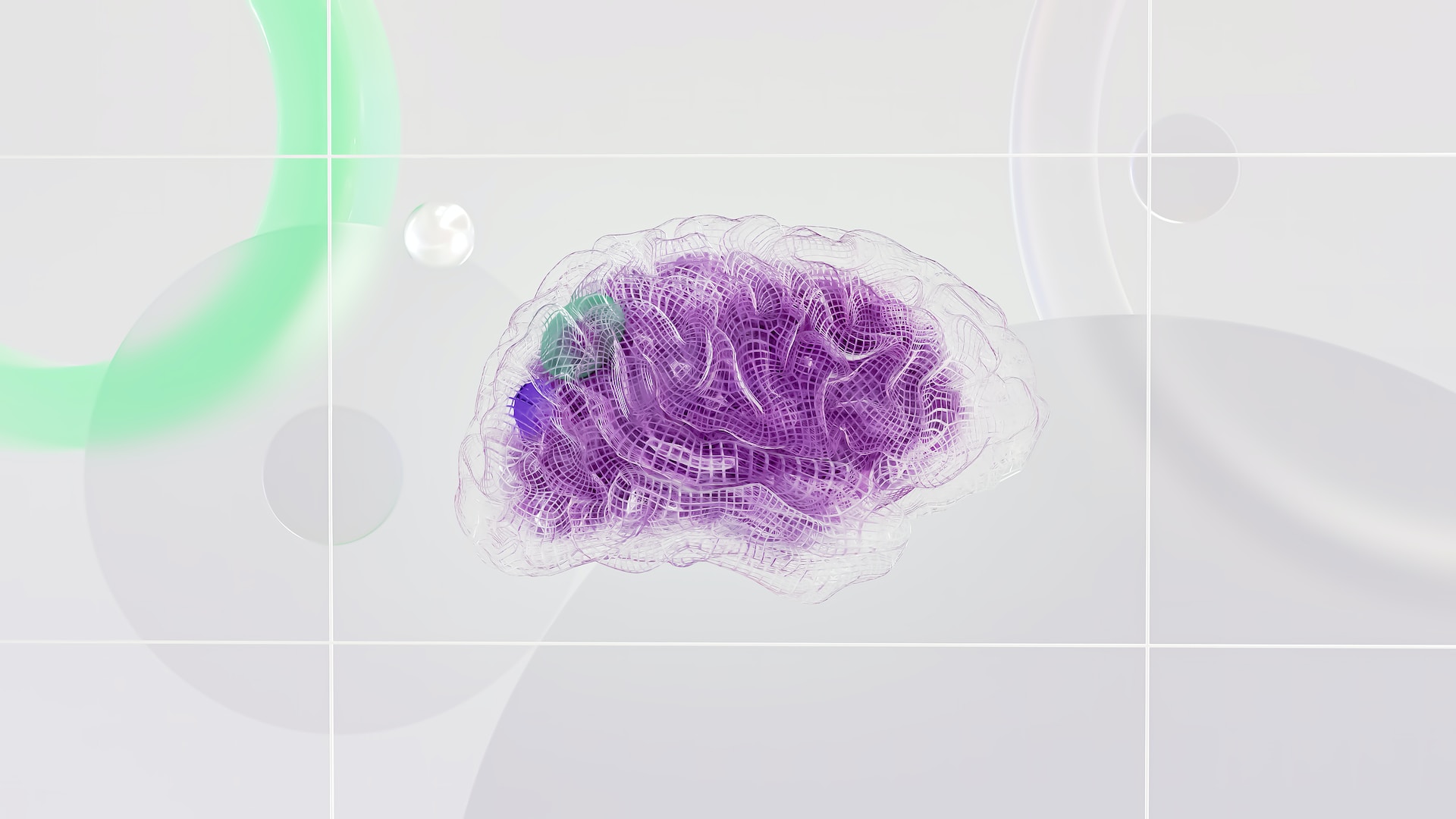
We rely on our senses to give us context for the world around us. If someone says “Thanks a lot” in a heavily sarcastic tone, for example, we can hear that they’re not really thanking us at all.
This is something AI models have often struggled with. But with multi-modal AI, that could change.
Introducing multi-modal machine learning (MMML)
Multi-modal AI has roots in cognitive science – that is, the study of the human mind. One critical insight from this area is that our brains use multiple modes of data, gathered via our five senses, to understand the world.
For instance, when we see a picture of a dog, we might read some text next to it that explains what breed it is. Or we may hear happy barks if we have the pleasure of petting one in person.
The tech takes this knowledge and applies it to the world of AI.
A traditional AI model works with one form of input, or modality, like text. It might give you a response based on the text you’ve typed in before, and then use a feedback loop to refine and improve its future responses.
MMML, meanwhile, ingests and processes data from multiple sources. Think images, text, speech, and algorithms.
MMML can take this info, analyse it, match it to keywords, and get a more rounded understanding of the world around it. This means it can look at a situation closer to how a human would. And it can give us more accurate predictions and outcomes as a result.
Multi-modal AI can outperform traditional AI models in several tasks, like image captioning and machine translation.
This is because it uses all available info rather than just relying on a single modality – making it more robust and accurate.
It’s no wonder Forbes has labelled MMML as the next logical step for businesses that use video and image applications.
Here are some of the most exciting ways to harness it.
Super-charge chatbots
Some of the world’s biggest companies are investing in MMML and using it with natural language processing (NLP) to improve their customer experience.
That includes United Airlines. Its travellers can now interact with its in-app voice assistant and change travel plans by chatting with its AI-powered assistant on the phone.
In fact, 70% of the organisation’s customer enquiries are now managed this way.
NLP technologies can detect vocal inflexions, like stress. This adds context to their processing so issues can be de-escalated appropriately.
Meta is also leading the industry with its MMML tech, named FLAVA. By pairing visual recognition with language understanding and multi-modal reasoning, the platform can reply to user queries, offering up images and info.
Ace text translation
MMML provides a serious step up from Google Translate – which often fails to understand vital context, misunderstanding words with multiple meanings (like ‘can’ and ‘fly’ in English).
You can use it to translate manga comics, for instance.
Researchers in Japan used MML with text analysis to translate the words in speech bubbles into Chinese and English. The tech does this using scene grouping, text ordering, and semantic extraction.
The result? Instant, automatic, and – perhaps most impressively – completely correct translations.
Find real-life risks
Short of hiring someone to sit and watch your warehouse operators on CCTV all day, there’s no way to make sure they’re working safely and to full capacity. Right?
Wrong. As MMML can integrate with computer vision technologies – for image and video capture, and object detection and recognition – it’s able to spot anomalies in real-life situations.
That means it can send warnings when it sees something risky, or integrate with Internet of Things (IoT) interfaces to send instructions to machinery – boosting productivity without the 1984-style monitoring.
That’s how Renesas Electronics and Syntiant are using the tech, anyway.
It’s also how it’s employed in the healthcare field – monitoring patients’ vital signs and diagnostic data – and in the automotive space – watching drivers for signs of drowsiness, like drifting out of lanes unexpectedly or their eyes being closed for extended periods.
The challenges of MMML
While MMML may seem like the stuff of data-science dreams, it isn’t without its setbacks.
Multi-modal AI is limited in:
- Finding a common language to communicate and translate data so it can be used
- The availability and expense of large, high-quality, diverse data sets
- Transferring knowledge between systems
- Associating data with predictions
Still, while the tech may have a way to go until it’s widely accepted, it’s certainly worth considering when researching and developing your models.