AI hallucinations: What they are and how to beat them
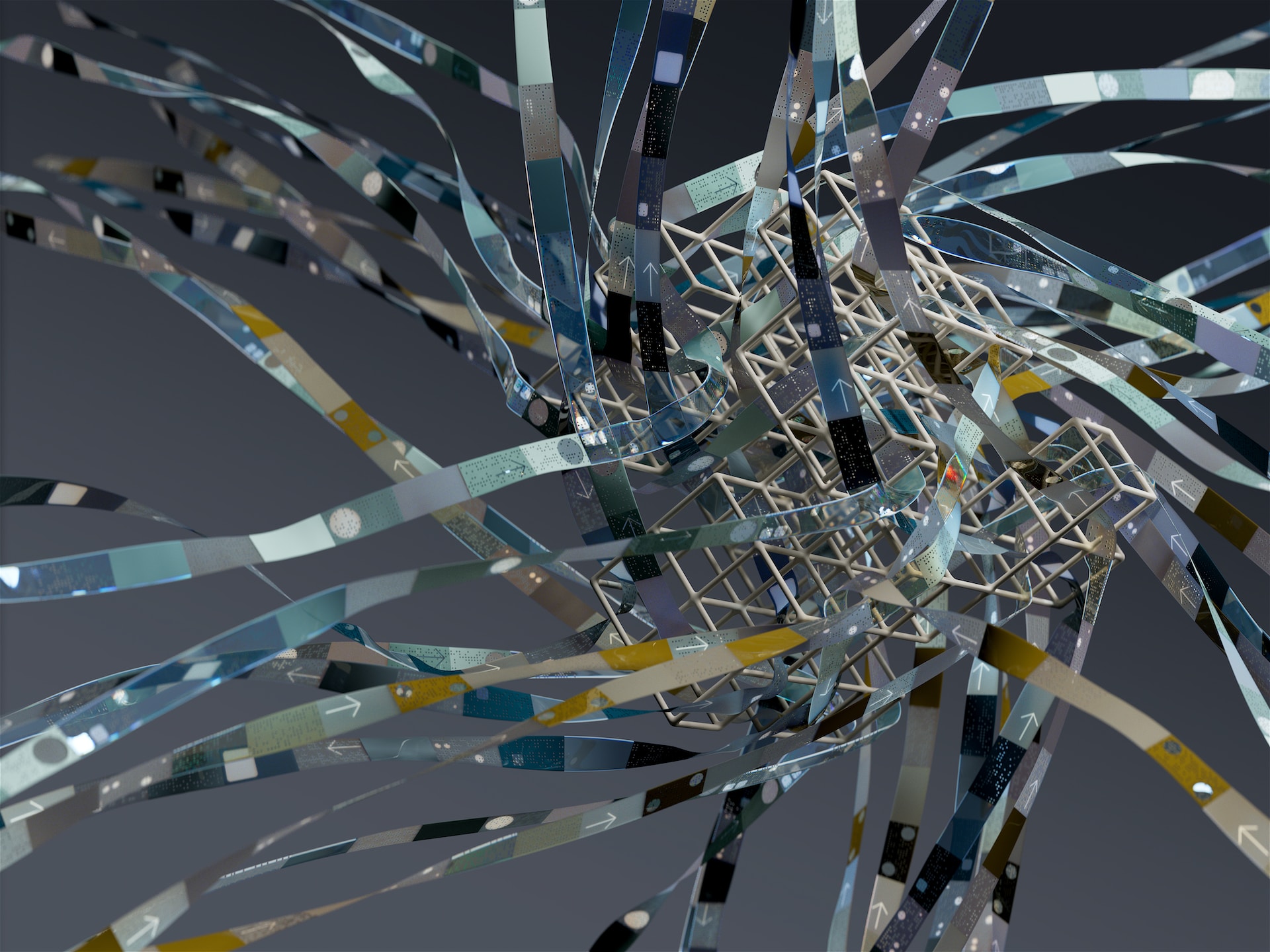
If you’ve tried your hand at creating AI art or using an artificial intelligence chat tool, you’ll know that both can produce some surprising – and, frankly, terrifying – results.
One anomaly you might’ve noticed is what’s known as AI hallucinations. What are they? And how can we remove them from AI models?
What are AI hallucinations?
AI hallucinations happen when an AI system, like a neural network, creates an output that’s seemingly unrelated to your inputs.
The result? Patterns, objects, or speech you weren't expecting – or that don’t even make sense.
This phenomenon results from the AI’s attempt to interpret and generate outputs based on the data it’s been trained on.
Let’s take it back to that AI-generated art we talked about. Crafting your own images with the help of AI tools has become increasingly popular, with algorithms producing a range of creative pieces.
We gave this a go ourselves, virtually painting a badger eating a pizza in the style of Rembrandt. The results were both hilarious and adorable – until we took away the Rembrandt reference, at which point it became a swirly scene of nightmares. Covered in melted cheese.
And we’re not alone in this horror. Many of these pieces have been known to contain seemingly random elements or patterns, which are not present in the original input. For example, a landscape painting might include a face. (Told you it was scary.)
In AI-generated text, hallucinations might look like words or phrases that are irrelevant or incoherent. A hallucinating chatbot with no training data regarding Tesla’s revenue might internally pluck a number out of thin air, such as ‘$13.6 billion’, that the algorithm ranks with high confidence.
The bot would then go on to falsely, repeatedly represent that Tesla’s revenue is $13.6 billion, with no provided context that the figure was a product of the weakness of its generation algorithm.
Another example of hallucination in AI is when a chatbot forgets that they are software and – alarmingly – claims to be human.
A quick note on language
Some experts oppose the use of the word ‘hallucination’, as it conflates the output of algorithms with human psychological processing.
In response to a disclaimer from Meta about their tool Galactica, linguist Emily Bender wrote:
“And let’s reflect for a moment on how they phrased their disclaimer, shall we? ‘Hallucinate’ is a terrible word choice here, suggesting as it does that the language model has experiences and perceives things. (And on top of that, it’s making light of a symptom of serious mental illness.) Likewise, ‘LLMs are often Confident’. No, they’re not. That would require subjective emotion.”
Another proposed term is ‘confabulation’ – though ‘hallucination’ is still the most used, which is why we’ve referenced it as such in this blog post.
How do hallucinations happen?
Some believe that when an AI creates a ‘hallucination’ while trying to figure out what’s in a picture, it might be doing the right thing based on what it’s learned.
For instance, if shown a picture that looks like a regular dog to us, the AI might pick out tiny details that usually only show up in cat pictures – essentially noticing things in the real world that we can’t easily see ourselves.
Then, it might pop a cat’s tail or whiskers into its final work, despite the fact you never mentioned cats (or wanted to include their features).
However, most AI hallucinations stem from limitations in the training data provided to the AI system. These limitations can result from:
Insufficient or biased data
If the dataset is limited or biased, the AI might struggle to interpret new inputs accurately.
Overfitting
AI systems may become too specialized in their training data, which makes it harder for them to generalize new inputs.
Lack of context
We see this when a model has limited understanding of different languages. Though a model may be trained on a vast set of vocabulary words in multiple tongues, it may lack the cultural history and nuance to string concepts together correctly.
Ambiguity
Ambiguous or ‘noisy’ input data can also confuse AI models.
Malicious attacks
That is, trainers with an ulterior motive deliberately manipulating the inputs to an AI model.
Complicated models
AI models with more layers or parameters may be more prone to generating hallucinations due to their increased complexity.
What can be done about them?
Guided refinement from real people is the key to helping with AI accuracy issues. This is known as human-in-the-loop (HITL) training.
HITL training sees human experts working with AI systems to review, correct, and provide feedback on AI-generated outputs.
This feedback helps make the AI system’s output more accurate and reliable.
Here are just some of the many reasons HITL training is essential for addressing AI hallucinations:
Quality control
In the first instance, human experts can help find and fix AI hallucinations. This ensures the AI system gives the accurate, coherent, and contextually relevant output people expect.
Battling bias
Involving human experts in the AI training process helps identify and address biases in the training data, leading to a more balanced and diverse dataset for AI systems.
Learning loops
HITL training allows AI systems to keep learning from human feedback, which makes them more adaptable and reduces overfitting.
Collaborative creativity
The partnership between humans and AI systems boosts both parties’ creative capabilities. Human experts can guide AI systems to create more relevant and aesthetically pleasing outputs, while AI systems can spark our imagination and help us find magic in the mundane. It’s a win-win.
Prolific is here to help
AI hallucinations present a fascinating and complex challenge that researchers must address as AI capabilities continue to expand.
After all, as the technology becomes increasingly integrated into our everyday lives, we simply can’t afford for it to make costly mistakes or spread convincing misinformation. And human feedback is key to helping us negate these possibilities.
At Prolific, we’re proud to have an engaged, enthusiastic, and ethically treated group of participants ready and raring to take part in AI tasks. In fact, this type of work is a favorite among our pool.
To find out more about how Prolific can help with HITL training, visit our dedicated AI page and send a message to our sales team today.