Your two-minute guide to Frequentist and Bayesian statistics
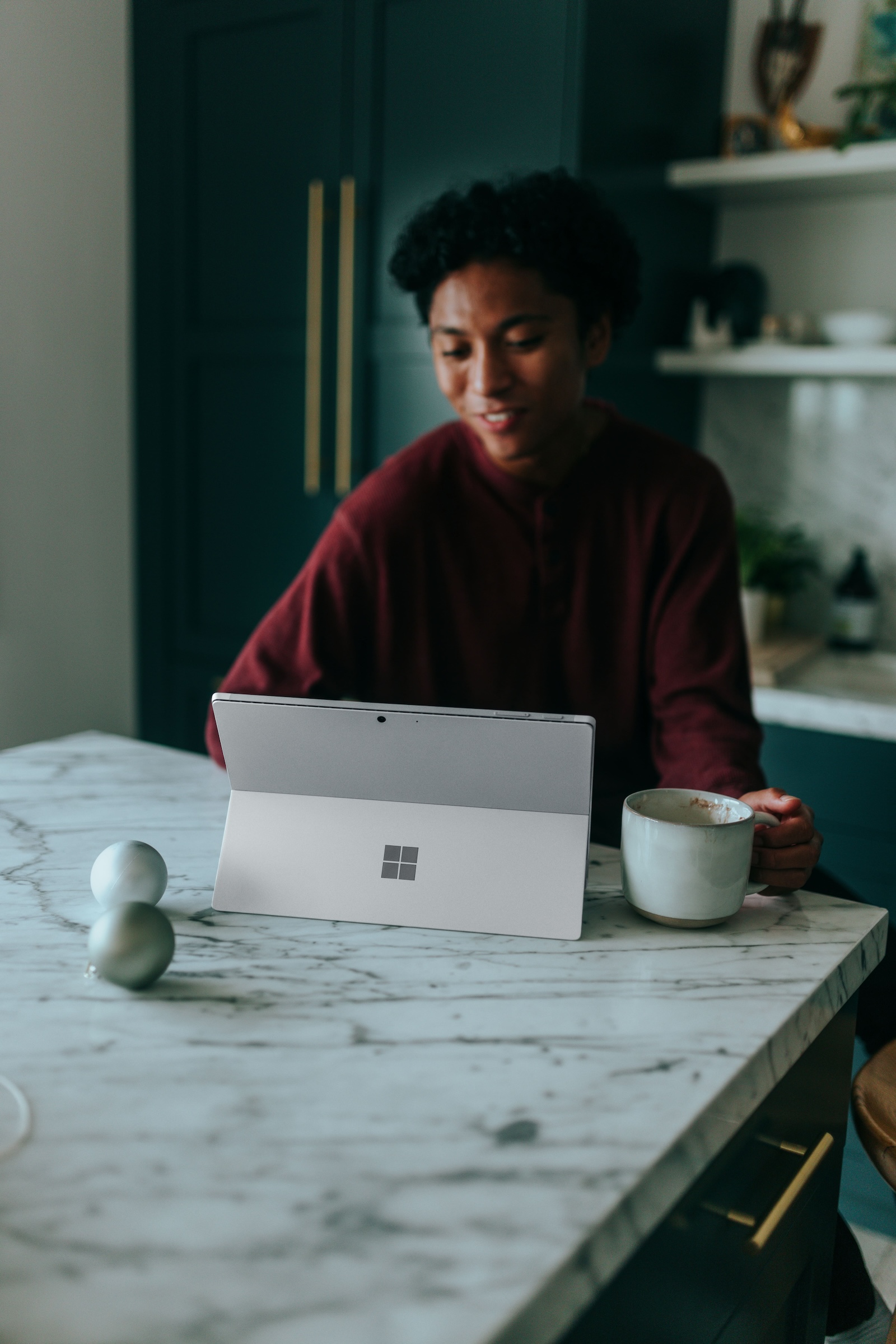
It’s a hotly debated topic in the research world – but one that’s often misunderstood.
We’re talking about Frequentist and Bayesian statistics: the two approaches to data analysis that can affect your interpretation of your results.
Here’s a quick overview of both that may just help you pick a preference. (Or understand the other better if you’re already engaged in the argument.)
What are Frequentist statistics?
By far the most implemented approach, Frequentist statistics – or Null Hypothesis Significance Testing (NHST), as it’s commonly known – involves setting up two hypotheses. These are the null hypothesis and the alternative hypothesis.
The null hypothesis states that there’s no difference or relationship between two variables, while the alternative hypothesis states that there’s a significant difference or relationship between two variables.
Null until proven alternative
Researchers using the Frequentist framework aim to find evidence that the null hypothesis is wrong instead of proving their alternative.
So, let’s say a study is being conducted into the correlation between height and shoe size. Our null hypothesis would predict that there’s no relationship between how tall or short people are, and how big or small their feet – while our alternative hypothesis would suggest a relationship.
After collecting our data, the Frequentist approach would see us trying to find evidence that our null hypothesis is incorrect; that there is, in fact, a relationship between height and shoe size.
The ever-elusive p-value
But how, you might ask? The answer: using a p-value.
And the problem: many researchers, despite their impressive training and years of experience, haven’t fully grasped what a p-value is. In fact, even Google’s got the definition wrong.
A p-value is often described as the percentage chance of the null hypothesis being correct. For example, if you had a p-value of .04, you’d have a 4% chance of your hypothesis being correct. Find a chair if you’re not already sat down; this isn’t the case.
In simple terms, a p-value represents the probability of seeing the pattern of data you collected if the null hypothesis was actually true.
Let’s say we had a p-value of .05 – the most frequently chosen threshold for ‘significance’, thanks to British polymath Robert Fisher – for our height vs shoe size study. This would mean that if there really was no relationship between the two variables, there would be a 5% chance of seeing the data you found.
Hackable stats
However, there are two issues with p-values.
Firstly, the more statistical tests you run (and the more p-values you create), the more likely you are to get a significant result simply by chance.
Secondly, and perhaps more worryingly, you can keep testing until you see something significant, and then report that test as if it’s representative. And by constantly running significance tests, smaller effects are more likely to appear significant, as your sample size increases, leading you to bias towards this significance.
What are Bayesian statistics?
Bayesian statistics offer an answer to the p-value situation.
With this approach, researchers look for evidence in favor of a hypothesis, not against it. And they do this using prior and posterior odds.
When you go into an experiment, you have some idea of what might happen – whether that’s a personal hunch or, ideally, an educated bet based on previous research or data.
This is what’s known as prior odds. These odds will be stronger or weaker depending on how much or little an inclination you have.
Coming back to our example, we might suspect that height is an indicator of shoe size. (One would hope that people are, on the whole, well-proportioned.) So, our prior odds indicate that we’ll probably find a correlation.
Before and after. And after, and after.
That’s where posterior odds come in. These are your actual odds of your hypothesis being true, based on evidence – so, after you’ve collected your data.
By gathering more and more data – and basing your findings on this data – your posterior odds essentially become your prior odds. Your initial guesswork becomes more refined as it updates in line with your evidence so far.
Let’s say we have a decent amount of data on people’s height and shoe size, and our data seems to indicate there’s, in actual fact, no relation between the two. As we move forward and collect more data, we go into this process knowing we likely won’t see the connection we initially predicted.
In the Bayesian framework, this likelihood of our hypothesis being true or false isn’t known as a p-number, but a Bayes factor.
So, which method is most accurate?
Of course, the big conundrum is, ‘which framework is best?’
Sadly, it isn’t an easy one to solve – especially not in a brief blog post like this – as both methods have their plus points and detractors.
We recommend you consider both, which you can do quickly and easily using JASP: a statistical program that’s free to download and use.
Whichever framework you end up choosing, the most important thing is that your work is as high-quality as possible. Our complete best practice guide to online research can help you rise to this challenge – offering tips for designing, piloting, and launching an effective study; advice for analyzing your data; and much more.
Download it for free, and get in touch with Prolific if you need any more assistance. We’ll empower you to run your research confidently, with satisfyingly fast, flexible, scalable studies powered by 100%-reliable data. (No p-value hacking here.)