It’s not how you test, it’s who you test: An interview with Kim Uittenhove
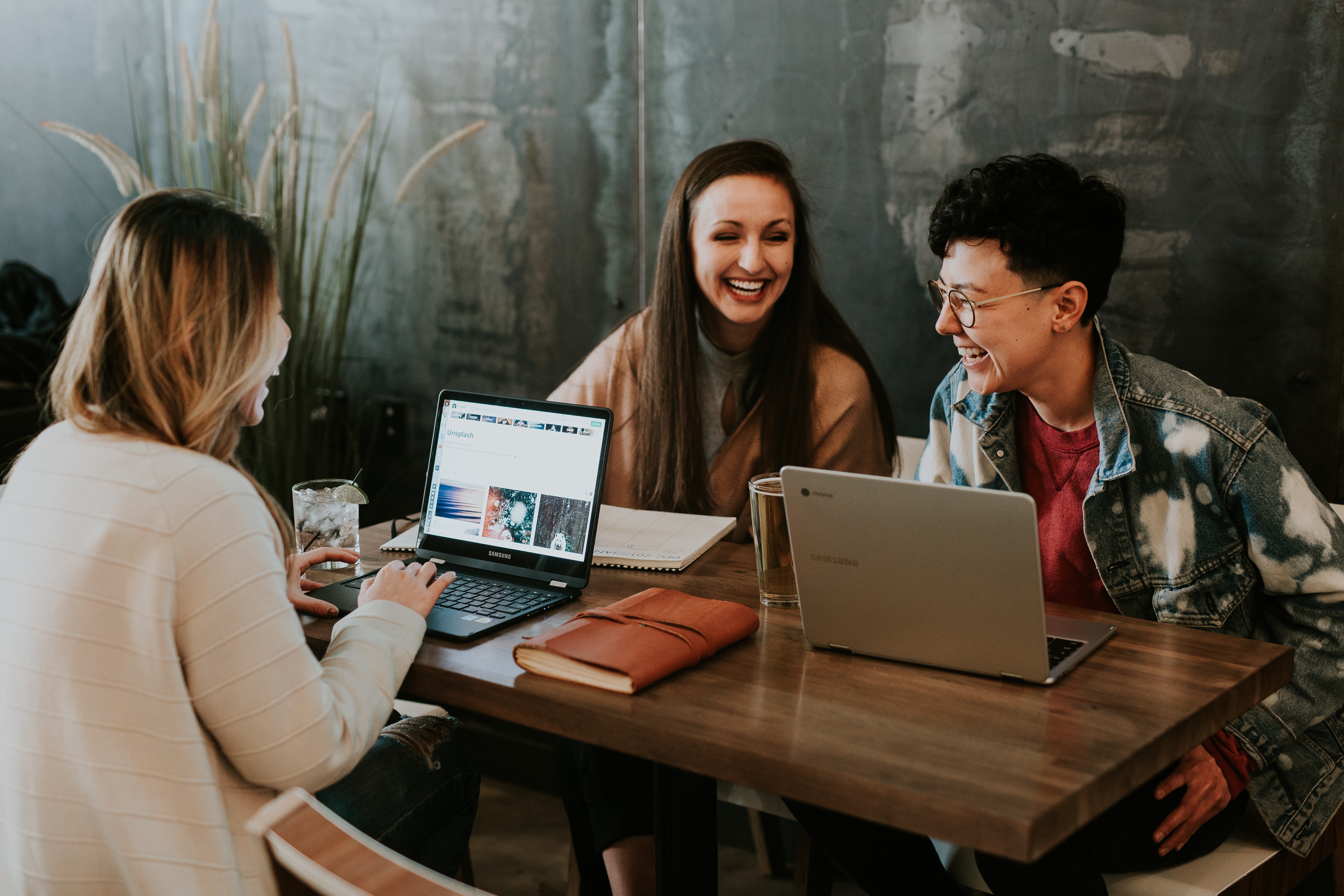
Data quality remains one of the top concerns that researchers have about online sampling and testing. Despite evidence to the contrary, there is a perception that the data quality from online samples is worse than the typical university samples used in research. However, no research has yet examined the impact of both how you test and who you test at the same time, until now.
I recently spoke to Dr. Kim Uittenhove from the University of Lausanne about her new paper in the Journal of Cognition that seeks to address this exact question: From Lab-Testing to Web-Testing in Cognitive Research: Who You Test is More Important than how You Test. You can read the full paper here.
Thanks for taking the time to talk with me today. Can you give us a brief introduction to yourself and your area of research?
I’m currently a Senior Researcher at the Institute of Psychology, University of Lausanne. I'm from Belgium originally, and I did most of my studies there at the University of Ghent, where I specialized in Theoretical and Experimental Psychology. This guided me towards a career as a cognitive scientist. During my PhD, I focused on cognitive aging, exploring various aspects of memory and cognition. Currently, I am studying cognitive aging in Swiss centenarians, as part of a large-scale project called SWISS100.
Besides my interest for cognitive research themes, I am also engaged in refining research methodologies, developing new paradigms, and enhancing data analysis strategies. I like to implement new and creative ways to conduct research.
Today we’re going to be discussing your paper, which is methodological in nature. Given that your background is in cognitive aging research, what got you interested in this other area of work and led to this paper?
The study was born from my own experience. I was working on a project that aimed to use machine learning algorithms to detect patterns in working memory task data. To achieve our aims, we needed a lot of data, which meant that our typical approach of testing participants in the lab was not going to work. It's very slow, costly, and also you're going to select a very specific group of people - students.
So, we started looking into options and the most obvious one at first was MTurk. It's fairly well known and it's been around for a while. Papers have used it successfully in the past. So we started implementing our study on MTurk.
Fortunately, I had implemented a data quality check to ensure that participants were actually engaging with the task, and not just pressing buttons while not paying attention. I noticed after getting about 100 participants that the rate of passing this data quality check was much lower than what I would have expected. This was concerning to me.
I started to wonder, is it just because our paradigm is a bit different from what is typically done on these online testing platforms? It asks for quite a bit more diligence on the side of the participants. This made me question - could the issues I was seeing be due to the fact that we were now using web-testing instead of monitored lab-testing?
So, we decided to expand the study to look at other participant pools and to also include a lab-testing modality. So, in addition to the original, intended research, we started working on a piece about data quality related to web-testing.
Do you know of any research that has directly assessed testing modality effects on data quality?
I could not find a study that disentangles the effects of which participants and which testing modality. Typically, in most research comparing online versus lab samples, there's a confound, because usually both the population and the modality changes, for example when comparing lab-tested students to web-tested MTurk participants. So it’s very hard to ascribe any observed differences to the fact that some participants were tested online versus offline.
Can you tell me how you designed the current study to address this issue?
We recruited four different groups of participants.
The first were students from the University of Geneva that came into the lab to participate, while being monitored. The second were students from the same pool who took part in the study online, without being monitored. The third was a Prolific sample, and the fourth was the MTurk sample, both of which also participated online without monitoring. This gave us two different testing modalities (web-testing and lab-testing) and three different participant pools (students, Prolific, and MTurk). Most importantly, students from the University of Geneva were enrolled as participants in both lab-testing and web-testing, allowing us to study the effect of using web-testing separately from the participant pool effect.
For each of the groups, we had participants complete a working memory task. Typically, in a working memory task, we present a person with stimuli to be remembered shortly after. In our case, these stimuli were letters - for example, BDCK. Then the person has to try to keep those letters in their memory so that we can test whether they remember the letters shortly afterward.
We tested memory for the letters by showing participants a letter and they had to indicate whether it had been part of the original set or not, by pressing one of two buttons. In our task, we also gave participants a distractor task in between seeing the letters and having to remember them.
How did you operationalize ‘quality’ in this study? What was an indicator of high vs low quality?
We measured quality in a few different ways. First, I looked for participants who had anomalous data patterns, for example when there was an unusually high number of extremely fast responses. Such responses are too quick to be considered genuine when participants are asked if they have seen a specific stimulus before. If a participant exhibited many such responses, our algorithm would flag that person as having an anomalous pattern.
Another measure of data quality consisted of verifying whether very well-known benchmark effects from the working memory domain would be present in these data. One such effect that we tested is the verbal disruption effect. This effect from the working memory domain shows that when people have to maintain a series of letters in their memory, but at the same time you’re asking them to repeat something out loud - in our case, this was saying “Mamma Mia, Mamma Mia” continuously - this verbal repetition should interfere a lot with their performance on the memory task, leading to much less correct responses.
So we have our data quality measures related to anomalous patterns, we have data quality measures related to the presence of important benchmark effects, and then we can compare these measures between testing modalities and between the different participant pools.
What were your findings?
The data quality for the lab-tested students was very good. They had few anomalous patterns and every single participant showed the verbal disruption effect. Then when we looked at the web-tested students, we saw that there was some decline in data quality. Specifically, a proportion of these participants did not show the verbal disruption effect.
This led to approximately 17% loss in data quality, which means that if you test students on the web, you would need to test around 20% more people. But I think this is offset by the convenience of web-testing. You don't need an experimenter to be present, you're getting your data faster, and you're saving a lot on all the other costs that come with in-person lab-testing. It’s a viable alternative, even for something as complex as the kind of memory task with specific instructions that we used in our study.
We also found that Prolific participants were almost indistinguishable from the web-tested students. They had very similar data quality according to all our metrics, so I think for researchers that want to branch out from using their own university student sample, Prolific seems like a very good choice and yields comparable results. In terms of demographics, the people who participated on Prolific had quite similar demographics to the people that we usually test in the lab, but of course, you can select different demographics if you want.
Then, at least with our specific paradigm, the performance of participants on MTurk was much lower than what we had on Prolific and for both groups of students. Only around 30% of MTurk participants passed all of the quality checks, which corresponded to a data quality loss of approximately 60% compared to participants in the lab. This was especially concerning when you understand that we applied the typical selection criteria that are usually advocated in research, which is to take a minimal approval rating of 95%, and a minimal number of 100 tasks already approved, which of course leads to the inclusion of more experienced people.
The take-home message is that web-testing is definitely a viable alternative in psychology research, even for more complex experiments like in our case. But you have to think about the platform that you use. I can definitely recommend Prolific to any researcher that wants to do this type of study.
Do you have any plans for future research on this topic?
At the moment, no, because I’m currently working on a project with centenarians, and that’s a population that would be difficult to test online as you can imagine. But in future research, if I'm doing online data collection again, data quality is something that I will think about upfront - and specifically which trackers I want to build in for data quality.
I think that in any case, it’s a good idea for researchers to keep communicating about data quality and sharing their experiences so that we have a better view of the current landscape.
Where can our readers follow your work?
The best place to follow my work is probably through my webpage at the University of Lausanne.
This research was carried out using the Prolific platform and our reliable, highly engaged participants. Sign up today and conduct your best research with our powerful and flexible tools.