The complete guide to improving data quality in online surveys
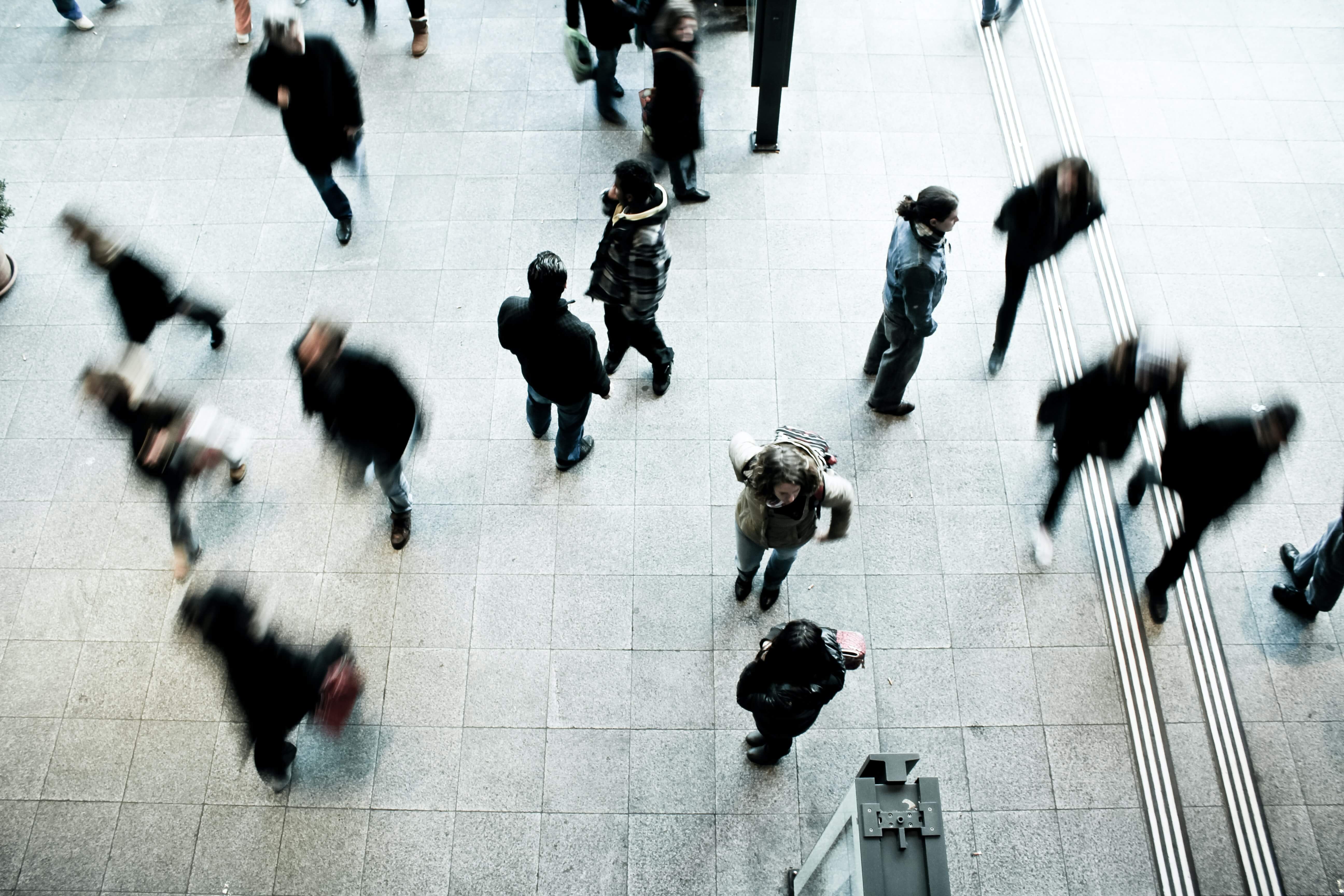
When it comes to data, “quality” can feel like an abstract idea — you don’t always know it when you see it. But it’s a big challenge for businesses. A 2021 survey of 300 data and analytics leaders across Europe and the U.S. found that 39% of respondents said data quality was a top challenge with using data to drive business value at their companies.
Whether you’re planning a new product line or a marketing campaign, online surveys and customer research are valuable sources of insights. They help you recognize when you’re onto a winner and when you need to rethink your options. But how can you tell if you’re getting high-quality data?
You need to be able to spot indicators of poor data-quality and the warning signs that point to poor-quality survey responses. Otherwise, you risk relying on poor-quality data filled with misleading responses that can steer you in the wrong direction — like launching products nobody wants or marketing campaigns that miss the mark.
What is data quality?
Data quality is a measure of whether data is fit for its intended purpose. It measures whether data will give you usable, accurate insights based on several different factors, including completeness and validity. Several common characteristics can help you identify high-quality data, and when you collect data via online surveys, there are some additional factors that are specific to this data collection process that can affect your data quality.
Comprehension
In online surveys, comprehension means whether or not respondents understand what you’re asking them. It’s an essential factor in data quality because if survey respondents don’t understand the questions, their responses won’t be accurate or honest.
Researchers often add instructions and a couple of preliminary questions at the start of the survey to check that the respondent understands their instructions. Even if they pass your comprehension check, you may still get low-quality responses from your participants if your survey design is difficult to understand.
Some ways your survey can affect comprehension include how you word your questions and the rating scales for responses. For example, researchers should make sure their questions are worded clearly to ensure there’s no room for misinterpretation when respondents answer them.
Attention
Attention is whether your participants are engaged with your survey and paying attention to what you’re asking instead of trying to multitask while watching Netflix. Respondents’ attention levels have a huge impact on the quality of responses you get to your online surveys because if they’re not paying attention, they’re more likely to misread questions or give minimal responses.
You can add attention check questions to your survey to check whether participants are fully engaged with the survey. Additionally, if you monitor how long respondents spend on your survey and on each question, you can spot when they’re paying attention compared with when they’re skimming through each question as quickly as possible.
Honesty
In online surveys, honesty is whether or not participants are giving truthful responses. It’s an essential part of data quality because dishonest responses skew your data and can lead you to draw misleading conclusions.
Sometimes, respondents may be tempted to tell you what they think you want to hear. For example, if a survey about workplace productivity asks how many times you visit social media sites each day, they may be tempted to respond with a smaller number than is true to appear more engaged with their work. Alternatively, you may be asking personal questions about private aspects of the respondents’ lives. If they feel like you’re prying, or if they don’t trust you’ll handle their responses confidentially, respondents are unlikely to be honest.
Accuracy
Accuracy is whether the data you collect matches reality. In online surveys, participant attention levels, comprehension, and honesty affect the accuracy of their responses. Respondents can accidentally enter inaccurate information if they don’t understand or are not paying attention or deliberately provide misleading responses if they're answering surveys dishonestly.
Accuracy is essential for data quality because inaccurate data skews your analysis. For example, if enough respondents choose the wrong age range (25-30 rather than 35-40), then your analysis of responses across age ranges will be skewed by those incorrect answers.
Completeness
Completeness is whether your data is complete, with all records present and fields filled in. In online surveys, completeness is measured by how many questions respondents have answered, which can be affected by participants not paying attention or not understanding the questions.
It impacts your data quality because incomplete data means you don't get all the information you need from survey respondents. For example, your survey data can be affected by completeness if respondents skip questions or leave one-word answers to open-ended questions. At best, it can leave you with gaps in your understanding, and at worst, it can skew your data if some of your questions go unanswered by a key subset of your target customers.
Consistency
Consistency is whether a participant answers the same question in the same way at different times in your survey. It affects your data quality because you can’t tell which is the accurate response.
Seemingly small inconsistencies can affect the accuracy of the responses you collect. For example, if you ask about employment status, and the respondent chooses “employed full time” and “self-employed full time” at different stages of your survey, you can’t tell which truly represents their work situation.
Reliability
Reliability is closely related to consistency, but it’s largely a psychological construct rather than a specific characteristic that your survey participants display. A reliable respondent will answer a survey the same way, no matter when they answer it or if they answer the same questions multiple times.
It impacts your data quality if you’re tracking and comparing results over time or if you’re running the same survey multiple times. Several factors can affect the reliability of your data, including changes to participants, the time you run the survey, and any changes to the survey itself.
Naivety
Naive survey respondents have no experience with your survey topic, questions, or the process of completing online research.
Participant naivety can affect your data quality on two fronts. First, if a respondent is already familiar with your research process or topic, their existing knowledge may affect the way they complete the survey and introduce bias into their responses. And second, if you have a truly naive respondent, it’s hard to know how trustworthy responses are and whether they understand the survey.
Representativeness
Representativeness measures how representative your data sample is of your target populations. If your survey responses don’t come from a representative sample of respondents, it can affect your data quality by introducing bias.
For example, if a company sends out an employee engagement survey, they want to be confident that they get responses representing employees’ viewpoints at all levels. But if the majority of responses come from senior executives, their data won’t be representative of their employee experience at all levels of the company.
Thoroughness
Thoroughness is the detail and depth participants go into when answering open-ended questions. It affects data quality because thorough responses are typically more detailed, so you get more insights and information to review from your respondents.
Some participants may provide the bare minimum information when responding to an open-ended question — just one or two sentences. But others may provide a paragraph or two, taking the time to fully explain their opinion or provide additional details.
Timeliness
Timeliness measures whether the data you’ve collected has been analyzed and used within an appropriate timeframe. It can affect your data quality because if there’s a long gap between collecting your data and analyzing those responses, the insights you gain may be out of date. For example, if you collect customer feedback about how they use your product but don't analyze it for six months, you may have new features or integrations which completely transform how people use your product but aren't reflected in your data.
Timeliness can be affected by your data handling and processing times, as well as the types of data you collect. Some data types are more affected by time delays than others — for example, if you ask respondents their date of birth, it won't change, but if you ask their age, it will.
Uniqueness
Uniqueness is whether there are duplications in your data. In online surveys, you should look out for the same person completing your survey multiple times, as well as duplicated responses from multiple participants. Duplicate survey responses can indicate fraudulent responses — such as in this example where the researcher received the majority of their responses from bots. However, duplicate responses aren’t always of poor quality. For example, if you have many multiple-choice questions, you're likely to see more similar responses than for open-ended questions.
The uniqueness of your responses will be affected by the type of data you collect and the questions you ask. For example, if you ask respondents what state or town they live in, it’s unlikely you’ll get all unique responses — but that’s not a problem.
Validity
Validity means that the questions you are asking actually measure the thing you are interested in. It’s affected by the wording of your survey questions and the format of responses.
For example, if you’re running a survey to discover the factors that influence employee engagement, then asking to what extent the respondents agree or disagree with the statement “I enjoy my work” won’t help you understand that.
Invalid data negatively affects your data quality because it doesn’t relate to what you’re trying to measure or understand.
Why is data quality important?
If you’re spending a lot of time and money collecting survey responses, it’s important that you get high-quality responses to justify your investment. The quality of the data you get from online surveys and research directly affects the value and insights you can gain from that research.
It affects your decision-making
According to McKinsey, “Without [data], there can be no digital transformation to propel the organization past competitors. There are no analytics driving new sources of revenue. Even running the basic business well isn’t possible.” But poor-quality data can be even worse than no data at all because it may give you false confidence in your actions and decisions.
Poor-quality data from online surveys can lead you to inaccurate conclusions or show misleading trends. When you make decisions based on that data, you're making choices based on incorrect conclusions, despite your best efforts to make data-informed decisions.
If you can generate high-quality survey responses from engaged participants, data becomes a great support to your business decision-making. For example, your online survey can help you choose the messaging for your new product line, avoid a poorly-timed marketing campaign, or identify advertising that resonates with your target audience.
It impacts your bottom line
According to Gartner, “Poor data quality destroys business value. [...] organizations believe poor data quality to be responsible for an average of $15 million per year in losses.”
Poor quality data from online surveys can affect a company’s bottom line in many ways. On the simplest level, it takes time and money to run online surveys, and if they bring in low-quality responses, you're wasting money. Poor-quality survey data can also mean that you miss crucial business opportunities, marketing trends, or customer insights because valid responses get buried in nonsensical or invalid responses.
Thankfully, good-quality survey data can boost your bottom line by enabling you to spot trends and opportunities you might otherwise have missed. If you can generate high-quality survey responses from engaged participants, online surveys are a worthwhile investment to support your decision-making.
How to improve data quality from online survey responses
When you’re collecting data from online surveys, you can do several things to boost the quality of data you get from your research participants. These steps will help keep participants engaged with your survey and encourage them to provide honest, accurate, and detailed responses.
Set up pre-screening requirements to recruit high-quality participants
Pre-screening requirements are a helpful tool for ensuring that the only people completing your survey fit the profile of your target audience. Many online research platforms allow you to pre-select different criteria your participants need to meet in order to qualify for your survey, such as:
- Age
- Gender identity
- Languages spoken fluently
- Employment status
- Lifestyle and interests
Pre-screening requirements help you get your survey in front of relevant people who meet the criteria you set. There are a couple of ways to approach them. You can set up pre-screening options for your online surveys based on lots of different demographic criteria so that only people who fit those criteria can join your survey.
Phrase questions to keep respondents engaged
The wording of your survey questions helps keep respondents engaged — or makes them “zone out” when going through your survey. If you ask several similarly phrased or structured questions in a row, it can cause a type of bias called habituation. This occurs when survey respondents get used to the kind of survey questions you’re asking, so they skim over the question (and their responses) rather than paying close attention.
To avoid habituation and keep respondents engaged, write varied questions and use different question types to create an interesting experience for respondents completing your survey. For example, rather than having a run of questions that all use a 1-5 rating scale, you could add a multiple-choice or open-ended question to stop respondents from skimming through the questions.
Add attention check questions (ACQs)
Attention check questions help you understand whether your survey participants are genuinely paying attention to what you're asking or just skimming through your survey. They assess how engaged respondents are with your survey and help to improve data quality by screening out disengaged participants.
Add ACQs at one (or more) points in your surveys to help you identify disengaged participants. An ACQ instructs respondents to answer a question in a specific way in order to check whether they’ve paid attention to the question. It shouldn’t leave room for interpretation. For example:
When asked for your favorite color, you must select green. This is an attention check.
What is your favorite color?
Blue
Red
Orange
Green
Yellow
Participants who fail at least two ACQs (for surveys more than five minutes long) should be disqualified from your survey to keep your quality of respondents high. However, some studies have shown that overusing ACQs can negatively affect data quality, so you shouldn’t rely on them as your sole method for boosting the quality of your survey responses.
Make personal questions optional (and anonymous)
To keep your responses honest and maintain high quality answers, make personal questions optional if they’re not integral to your research. Many research platforms (including Prolific) don’t allow you to collect personally identifiable information, which maintains the privacy and anonymity of your respondents.
Personal questions can be a sticking point for survey respondents if they don’t feel comfortable answering them. This can lead to them providing false or nonsensical responses so they can move on to the next part of your survey.
At the start of the survey, let participants know that their responses are anonymous. Then remind them again when you get to the personal or sensitive questions. This will build trust and help them feel more comfortable sharing personal or potentially sensitive information.
Compensate respondents fairly for their time
One of the best ways to recruit high-quality survey respondents is to pay them fairly for their time. Doing so incentivizes participants to provide high-quality responses and take their time to answer your survey fully, rather than rushing to complete it as quickly as possible.
In the past, it was thought that paying survey respondents would negatively impact their responses and skew your data (as respondents would give the answers they thought you wanted). However, this is an outdated view, and almost all research participants are compensated for their time. Pre-screening respondents and adding attention checks can mitigate that risk, allowing you to recognize the valuable contribution your respondents make to your survey.
High data quality starts with high-quality research participants
The best-planned research in the world will still produce poor quality data if all your responses come from bots or disengaged respondents. Being able to recruit high-quality participants lays the foundation for collecting quality data from your survey. Learn more about the factors that matter most when recruiting high-quality survey participants — whether you’re using Prolific or any other online research platform.
Log in or sign up to Prolific today and launch your study to thousands of participants in minutes